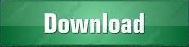
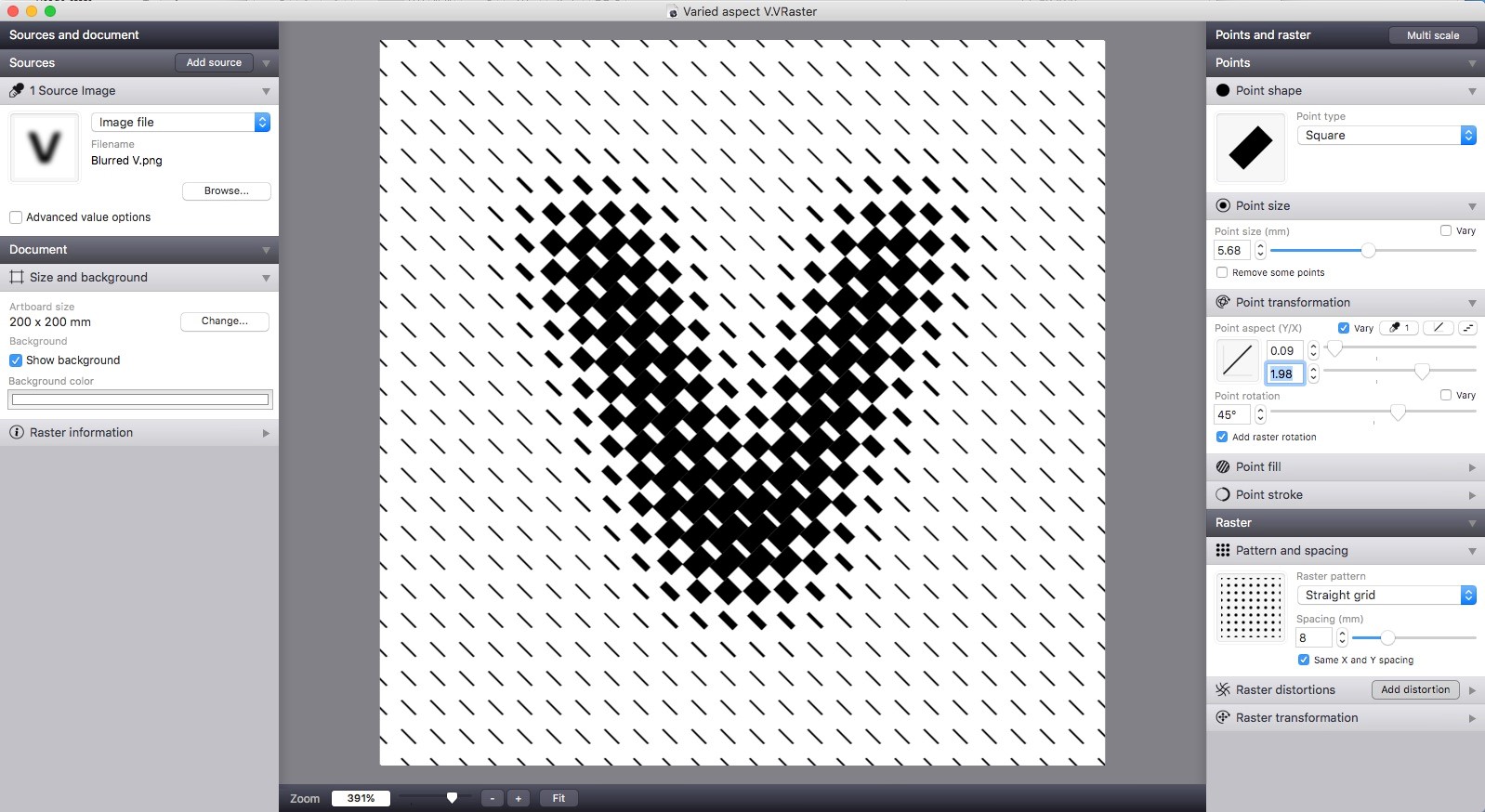

Identify Trouble Areas using Unsupervised Classification To reduce the potential number of misclassifications, the area covered by ocean was excluded from the image using the Extract by Mask tool and the outline of the island created in the previous post.
#Raster image procesor software
Initially I tried to classify the entire image, but reflections in the water and waves breaking on shore made it difficult for the software to construct an accurate classification, even using hundreds of training areas. An excellent resource on classification in ArcGIS10.0 is available here.Ī high quality vegetation classification can be a powerful tool in vegetation and habitat analysis , as it can provide a lot of information about a large area with relatively little work. In this final section, I’ll focus on classification techniques including identifying areas requiring more focused training areas, what makes a good training area, and how you can quickly and easily clean up your classified image. The Georeferencing and mosaicking of the imagery were covered in previous posts, as was creating a polygon mask of the island. The final goal of the project was to produce a detailed classification of the island’s vegetation from a series of digital aerial photographs. These images are from a project I recently completed looking at the structure of a seabird colony off the coast of Nova Scotia, Canada, and are representative of the less-than-ideal imagery many of us have to work with regularly. This is the fourth in a series of blog posts that will cover some tips and tricks for performing the following operations on a series of aerial images using ArcGIS 10.0:
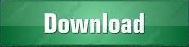